Researchers are exploring new methods for large language models (LLMs) to enhance efficiency and reasoning by allowing them to process information in mathematical latent spaces instead of converting everything into language. Current LLMs waste computational resources transforming latent representations into words, which can lead to information loss. Two emerging models, Coconut and a recurrent transformer, show promising results by enabling reasoning directly in latent space. While Coconut demonstrated improved efficiency over traditional models, both new approaches indicate potential for better performance albeit with some limitations. This shift could fundamentally change AI reasoning methods.
To Make Language Models Work Better, Researchers Sidestep Language
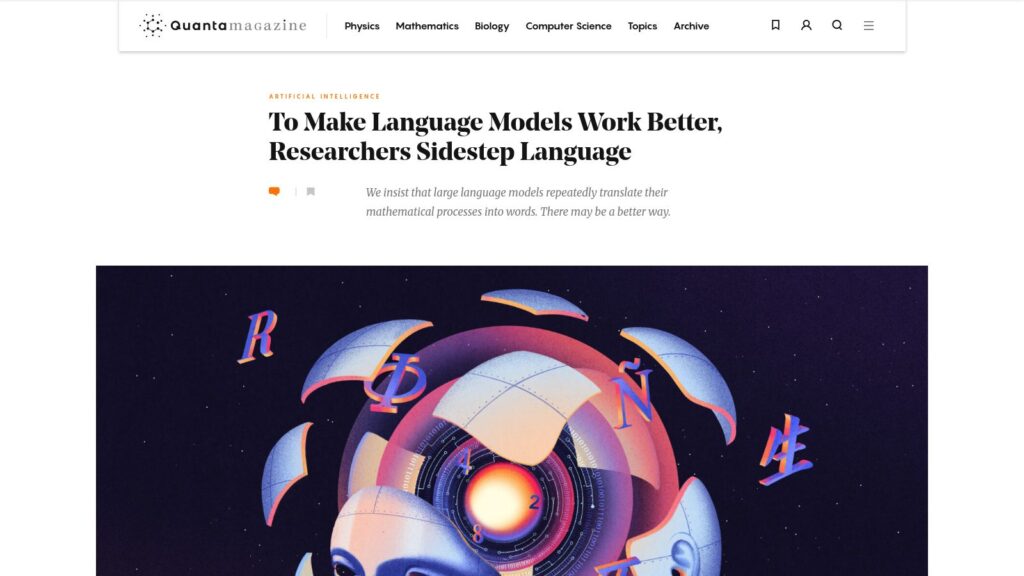